Knowing the presence or the actual number of occupants in a space at any given time is essential for the effec-tive management of various building operation functions such as security and environmental control (e.g., lighting, HVAC). In the past, motion detection using Passive In-frared (PIR) sensors has been widely deployed in com-mercial buildings and can provide data on “presence” sta-tus. However, there are known limitations with PIR sen-sors, even for occupant presence detection, in that detec-tion error can occur when the occupant is stationary or performing common tasks in the office space involving small movement such as typing or reading. Moreever, PIR can not detect the number of the occupants in the space.As occupants “interact” with the indoor environment, they will affect environmental conditions through the emission of CO2, heat and sound, and relatively little effort has been reported in the literature on utilizing this environ-mental sensing data for occupancy detection. This pa-per presents the findings of a study conducted at the In-telligent Workplace (IW) at Carnegie Mellon University (CMU) to address this question by exploring the most effective environmental features for occupancy level de-tection. A sensor network with robust, inexpensive, non-intrusive sensors such as CO2, temperature, relative hu-midity, and acoustics is deployed in an open-plan office space in the IW. Using information theory, the physical correlation between the number of occupants and vari-ous combinations of features extracted from sensor data from a 10 week period is studied. The results show sig-nificant correlation between features extracted from hu-midity, acoustics, and CO2, while little correlation with temperature data. Using features from multiple sensors increases correlation further, and over 90% information gain is acquired when at least six of the most informative features are combined. This work provides a foundation for future studies on using ambient environmental sensor data for occupancy detection.
Information-theoretic environmental features selection for occupancy detection in open offices
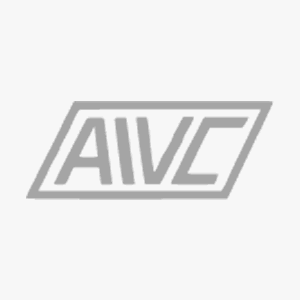
Year:
2009
Bibliographic info:
Building Simulation, 2009, Glasgow, Scotland