The objective of this work is to train an artificial neural network (ANN) to learn to predict the required heating load of buildings with the minimum of input data. An ANN has been trained based on 250 known cases of heating load, varying from very small rooms (1-2 mP2P) to large spaces of 100 mP2P floor area. The type of rooms varied from small toilets to large classroom halls, while the room temperatures varied from 18C to 23C. In addition to the above, an attempt was made to use a large variety of room characteristics. In this way the network was trained to accept and handle a number of unusual cases. The data presented as input were, the areas of windows, walls, partitions and floors, the type of windows and walls, the classification on whether the space has roof or ceiling, and the design room temperature. The network output is the heating load. Preliminary results on the training of the network showed that the accuracy of the prediction could be improved by grouping the input data into two categories, one with spaces of floor areas up to 7 mP2P and another with floor areas from 7 to 100 mP2P. The statistical RP2P-value for the training data set was equal to 0.988 for the first case and 0.999 for the second. Unknown data were subsequently used to investigate the accuracy of prediction. Predictions within 10% for the first group and 9% for the second were obtained. These results indicate that the proposed method can successfully be used for the estimation of the heating load of a building. The advantages of this approach compared to the conventional algorithmic methods are (i) the speed of calculation, (ii) the simplicity, and (iii) the capacity of the network to learn from examples and thus gradually improve its performance. This is done by embedding experiential knowledge in the network and thus the appropriate U-values are considered. Such an approach is very useful for countries where accurate thermal properties of building materials are not readily available.
Building heating load estimation using artificial neural networks
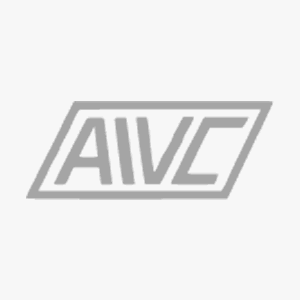
Year:
1997
Bibliographic info:
Belgium, Proceedings of Clima 2000 Conference, held Brussels, August 30th to September 2nd 1997