A building energy management system (BEMS) generally monitors and manages energy usage in commercial buildings. With the ability to monitor a plant and to recall the collected data at a later time, actual building energy performance can be measured and compared with the expected performance. The comparison will help in detecting possible abnormalities with the building energy usage and in identifying opportunities to optimize the building energy performance. In order to predict expected building energy performance, a reasonably accurate building energy model is needed. The building energy model is complex, multi-dimensional, and nonlinear. The relationship between building occupation and environmental conditions and the whole building energy usage can be established by using nonlinear models, e.g., artificial neural networks. There are many publications on using neural networks to estimate the energy usage. Most of these studies on load estimation are, however, based on one single data set. For such a neural network model to be commercially attractive, the model must be directly applicable to any building without any major design adjustments. The objective of this study is to design a neural network model that is generally applicable without major design adjustments and, at the same time, provides an estimation that is accurate enough for commercial purposes. This paper consists of two parts. Part I discusses various neural network methods, their design issues, benefits, and limitations. The discussions create a framework for selection of neural network models and their application to real building data, which is covered in Part II, along with results and analysis.
Development of a generalised neural network model to detect faults in building energy performance - Part 1.
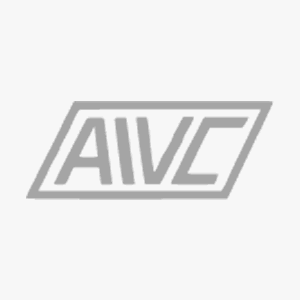
Year:
2000
Bibliographic info:
in: ASHRAE Annual Meeting 2000, proceedings of a conference held Minneapolis, USA, June 24-28, 2000.