The project aims to investigate the degree of influence that outdoor conditions may have on the indoor environment in Norwegian schools. It also aims to ascertain whether it is possible to use outdoor parameters such as particulate matter, relative humidity, and air temperature, along with indoor parameters including CO2, relative humidity, and air temperature, to predict indoor particulate matter values. The outdoor data was gathered from various weather stations near the schools, while the indoor data was provided by N3, who collected it using sensors within the schools. To predict indoor particulate matter values, a machine learning algorithm, random forest regression, was employed. The project's findings highlight the significant impact of outdoor conditions on indoor environments. These encompass a wide spectrum, ranging from local weather effects, like cold temperatures leading to pollutant accumulation at lower altitudes, to remote occurrences, such as Sahara sands traveling thousands of kilometres by wind to Norway. Variations in the correlation between indoor and outdoor PM10 values across different locations and classrooms suggest potential diverse sources of particulate matter, seasonal effects on indoor air quality, or disparities in ventilation systems and cleaning procedures. These initial findings were further investigated by a random forest regression algorithm in machine learning. This approach incorporated diverse outdoor and indoor parameters to assess feature importance and forecast indoor PM10 levels, resulting in robust models with achieved R-squared values reaching 0.92. In January, outdoor temperature emerged as the primary influential factor, followed closely by outdoor PM2.5 values and indoor relative humidity. In contrast, September emphasized indoor relative humidity as the most significant influence. Notably, indoor CO2 demonstrated a consistent level of influence in both January and September, likely due to consistent student activity. The robustness of the random forest models and their close alignment with actual PM10 values suggest a strong potential for establishing future cleaning procedures based on predictive models.
The Influence of Outdoor Conditions on Indoor Air Quality: Case Study of Norwegian Schools
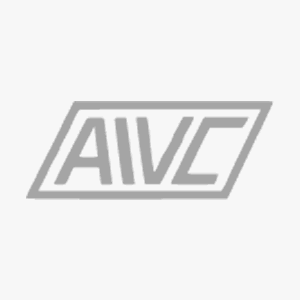
Year:
2024
Languages: English | Pages: 11 pp
Bibliographic info:
44th AIVC - 12th TightVent - 10th venticool Conference – Dublin, Ireland - 9-10 October 2024