Heating, ventilation and air conditioning systems, e.g., air handling units (AHUs), play a key role in modern building energy systems (BES) as they account for a high share of the energy usage, e.g., 30 % within the U.S. commercial building sector. Unfortunately, many faults occur during the operation of AHUs leading to higher energy usage and the need for expert personnel monitoring the according plants. The latter is a time-consuming task and is assisted by fault detection systems nowadays. While there are many different approaches to detect faults in air handling units, this paper presents an approach based on semantic knowledge.
For this approach, an ontology for fault detection and diagnosis (FDD) in AHUs is developed. The FDD ontology describes which components, e.g., temperature sensors or valves, can face what kinds of faults. Hence, the FDD ontology contains generic knowledge and is interoperable and reusable. Furthermore, generic semantic fault rules based on the air handling performance assessment rules (APAR) are developed.
For this generic concept, the actual information about the examined plants, their configuration, sensors, actuators, and controls are stored in a knowledge graph based on existing ontologies, like Brick Schema.
For the evaluation of the fault rules, the configuration of the plants is queried and rules get activated if the necessary components needed for evaluation of the rules are present. Afterwards, the according time series data for rule evaluation are fetched for analysis, following the linked data principle - in this work from a cloud platform. Depending on the analysis result, fault messages are gathered in a list and sorted according to their incidence to allow a certain prioritization for maintenance personnel. The fault notation follows a unified schema allowing personnel to understand and locate the faults fast.
The developed approach is validated using historical data of a real AHU on our premises. We inject faults into the historical data, e.g., drift, stuck, bias, fouling, abnormal, and frozen. The results indicate a high rate of fault detection, e.g., average accuracy of 99.3 %, precision of 97.85, sensitivity of 95.6 %, and Matthews Correlation Coefficient of 98.4 %. Therefore, the approach is considered valid for fault detection in AHUs.
In the future, it is planned to implement more complex faults, e.g., valve needle separated from drive or control faults. Furthermore, the semantic enriched plant configuration shall be used to improve the discovery of fault-symptom relationships.
The presented approach has the big advantage that the knowledge about air handling units and their faults needs to be formulated only once, leveraging semantic knowledge. Therefore, this generic solution can be used for diverse AHU configurations potentially reducing FDD development efforts.
Semantics-based expert system for fault detection in air handling units
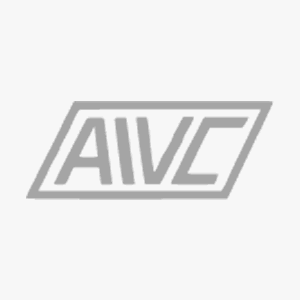
Year:
2024
Languages: English | Pages: 10 pp
Bibliographic info:
44th AIVC - 12th TightVent - 10th venticool Conference – Dublin, Ireland - 9-10 October 2024