This paper introduces a new approach for the prediction of hourly energy consumption inbuildings. The proposed method uses nonlinear timeseries analysis techniques for thereconstruction of energy consumption timeseries and the estimation of the dynamicinvariants, and artificial neural networks as a nonlinear modeling tool.Among the several neural network modeling factors that affect time-series prediction, themost important are the window-size and the sampling lags for the data. Relevant theoreticalresults related to the reconstruction of a dynamical system are analyzed and the relationshipbetween a correct embedding dimension and network performance is investigated.The problem is examined initially for the univariate case and is extended to include additionalcalendar parameters, in the process of estimating the optimum model.Different network topologies are considered, as well as existing approaches for solving multistepahead prediction problems. The performance of short-term predictors is also examinedwith regard to prediction horizon.The performance of the predictors is evaluated using measured data from real scalebuildings, showing promising results for the development of accurate prediction tools.
Short-Term Prediction of Energy Consumption in Buildings Based on Artificial Neural Networks and Nonlinear Time Series Analysis
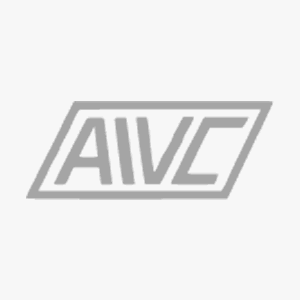
Year:
2006
Bibliographic info:
27th AIVC and 4th Epic Conference "Technologies & sustainable policies for a radical decrease of the energy consumption in buildings", Lyon, France, 20-22 November 2006